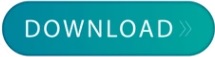


lel, for B steps, sampling from Multinom(w) – only Non-free implementation in Hom4PS-3. In the case of a polynomial system, the polyhedral homotopy method finds all the solutions (it – Metropolis: run N identical Markov chains in paralscales better than Gröbner bases).

– Rejection sampling, if an upper bound on the weights Homotopy continuation is a way of numerically finding the solutions of a system of equations, by folis known: lowing a homotopy from an easy-to-solve system to the j ∼ UnifJ1, N K while Unif(0, 1) > w1 /wmax desired one. (2015) The propagation and weighting steps of sequential Monte Carlo (SMC, particle filters) are easy to parallelize, but the resampling step is less so – try:įixed points of belief propagation an analysis via polynomial continuation C. The monomials xk are well-suited to find roots on the circle for roots on, prefer Chebychev polynomiParallel resampling in the particle filter als. The normative axioms of “social welfare theory”, non-dictatorship, universality, transversality, Pareto efficiency and independence to irrelevant alternatives (Arrow’s impossibility theorem) can be relaxed and satisfied. Distributional rank aggregation only uses the distribution (histogram) of the rankings. Rank aggregation is the problem of combining several rankings (e.g., from search engines) into one. i̸=j Distributional rank aggregation and an axiomatic analysis A. The restricted NMF requires rank M = rank W, ℓ′ (xj ) = (xi − xj ) defining a restricted nonnegative rank. The learning rate α should satisfy αt = ∞, αt2 rank+ in general (in dimensions beyond ∏ 3). This is off-policy learning: the first term comes from the policy actually followed (e.g., ε-greedy), the second from the optimal∑ policy found∑so far. target target = R(s, a, s′ ) + γ Max Q(s′, a′ ) ′ a.If the MDP is not known, we can act at random and estimate the expected Q-value (tabular Q-learning). Policy iteration alternates two steps: – Compute the value V π of the policy πk – Improve the policy by taking the best 1-step action πk+1 (s) = Argmax P (s′ |a, s) R(s, a, s′ ) + γV π (s′ ) aĢ. If the policy π is fixed, however, it is just a linear system, which can be solved iteratively or directly (policy evaluation). These are just systems of equations but, because of the maximum, they are not linear. In a duelling DQN, the neural network adds structure ∑ to the Q-value and separately forecasts state value and ′ ′ Qk+1 (s, a) = P (s′ |a, s) R(s, a, s′ )+γ Max Q(s, a ) advantage, Q(s, a) = V (s) + A(s, a). Q-value iteration is similar, but computes Q(s, a), a the expected value, if we start in state s, take action a, and act optimally thereafter. Double DQN uses two sets of weights, to select the best action, and to estimate it (otherwise Maxa Qθ (s, a) is biased upwards) Prioritized experience replay uses the Bellman error as transition weights. Neural fitted Q-iteration is a batch version of DQN: generate a lot of ε-greedy episodes fit the resulting target for a while iterate. – Use Huber loss instead of square loss – Use RMSProp instead of standard SGD – Anneal the exploration rate. Value iteration iterates the Bellman equation V0 (s) = 0

Planning or optimal control is the search of the optimal policy on a known Markov decsion problem (MDP).
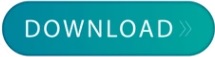